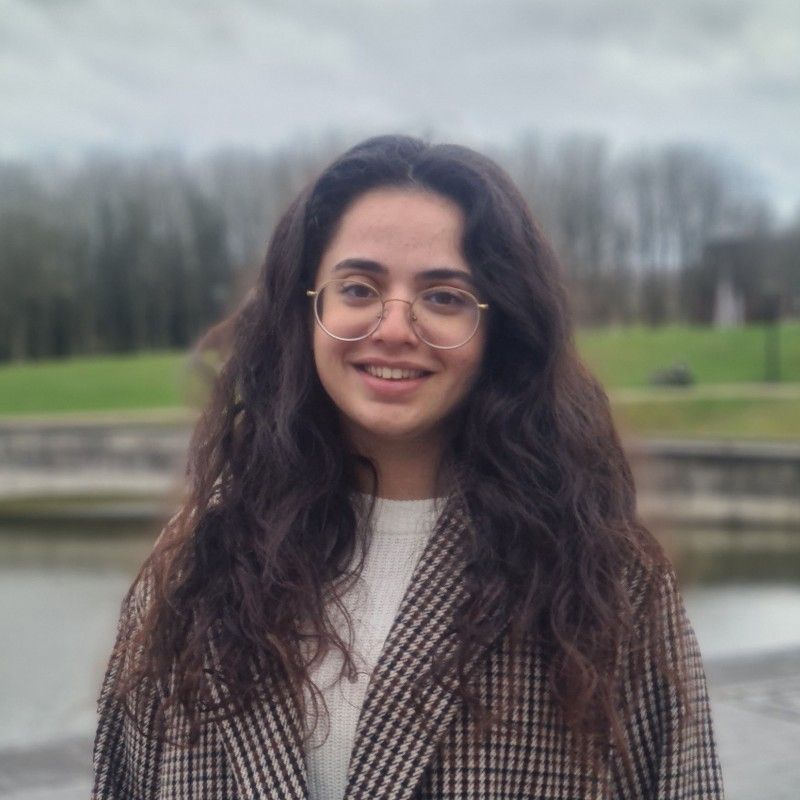
Carla Geara
Image Processing Engineer
We've developed InSAR2InSAR, a breakthrough self-supervised method that significantly improves the quality of InSAR data for forest monitoring without requiring noise-free data. By denoising interferometric parameters directly from real satellite imagery, our innovation enables more accurate assessments of forest structure, biomass, and carbon stocks. Unlike traditional approaches that rely on synthetic data, InSAR2InSAR leverages overlapping Sentinel-1 satellite observations to train directly on real-world conditions, resulting in superior performance across diverse landscapes. This advancement represents a significant step forward in our remote sensing capabilities for environmental monitoring and nature-based solutions.
Interferometric Synthetic Aperture Radar (InSAR) has emerged as a powerful tool for monitoring Earth's surface, with significant applications in forest structure estimation (Treuhaft et al., 1996). However, the quality of InSAR parameters, coherence and phase, is often compromised by speckle noise, limiting their practical utility in forestry applications. At Kanop, we have developed a new innovative self-supervised method, InSAR2InSAR, which effectively denoises these critical parameters without requiring clean training data.
Most existing denoising approaches for InSAR data, e.g. Φ-Net (Sica et al., 2021) and InSAR-MONet (Vitale et al., 2022), rely on supervised learning, requiring pairs of clean and noisy samples for training. Since obtaining clean InSAR data is practically impossible, these methods typically train on synthetic data, which cannot fully capture the complex properties of real-world images. This limitation becomes particularly problematic when applying these methods to forest environments, where accurate parameter estimation is crucial.
InSAR2InSAR extends the Noise2Noise (Lehtinen et al., 2018) principle to the joint estimation of InSAR parameters. Rather than requiring clean samples, our method leverages two independent noisy observations of the same scene, allowing us to train directly on real data. The key insight is that when the expectation of these independent noisy observations matches the clean target, we can train a network to denoise effectively by mapping one noisy sample to another.
What makes our approach unique is that we focus on denoising the interferometric covariance matrix, which encodes both coherence and phase information. By estimating this matrix, we simultaneously recover both parameters with improved quality.
Initially tested on simulated data (Geara et al., 2024), InSAR2InSAR demonstrated superior performance compared to state-of-the-art supervised methods like Φ-Net and InSAR-MONet. The real breakthrough, however, came with our discovery that Sentinel-1 satellite data, specifically the burst overlaps in Interferometric Wide Swath mode, fulfill the requirements of Noise2Noise, providing the perfect setup for training InSAR2InSAR on real data (Geara et al., 2025). Tests on real Sentinel-1 data across diverse European landscapes confirmed the method's effectiveness, with significantly reduced noise levels and preserved structural details.
Forest structure estimation from remote sensing data requires high-quality parameters that accurately represent the complex structures of forest ecosystems. By providing cleaner coherence and phase estimates, InSAR2InSAR enables more reliable extraction of forest height, density, and biomass information, critical variables for forest management, carbon stock assessment, and biodiversity monitoring.
The reduced noise and improved signal quality mean that even in areas with dense vegetation, where coherence is typically low and phase information is noisy, InSAR2InSAR can recover meaningful parameters that better correlate with forest structural attributes.
The benefits of InSAR2InSAR extend to other domains as well. Applications such as land cover classification, ground deformation monitoring, and earthquake analysis also depend on high-fidelity interferometric data. The ability of InSAR2InSAR to generalize across different landscapes makes it a powerful tool for a wide range of environmental and geophysical applications.
R. N. Treuhaft, S. N. Madsen, M. Moghaddam, and J. J. van Zyl, “Vegetation characteristics and underlying topography from interferometric radar,” Radio Science, vol. 31, no. 6, pp. 1449–1485, 1996, doi: 10.1029/96RS01763.
F. Sica, G. Gobbi, P. Rizzoli, and L. Bruzzone, “Φ-Net: Deep Residual Learning for InSAR Parameters Estimation,” IEEE Trans. Geosci. Remote Sensing, vol. 59, no. 5, pp. 3917–3941, May 2021, doi: 10.1109/TGRS.2020.302042.
S. Vitale, G. Ferraioli, V. Pascazio, and G. Schirinzi, “InSAR-MONet: Interferometric SAR Phase Denoising Using a Multiobjective Neural Network,” IEEE Transactions on Geoscience and Remote Sensing, vol. 60, pp. 1–14, 2022, doi: 10.1109/TGRS.2022.3224303.
J. Lehtinen et al., “Noise2Noise: Learning Image Restoration without Clean Data,” in Proceedings of the 35th International Conference on Machine Learning, PMLR, Jul. 2018, pp. 2965–2974.
C. Geara, C. Gelas, L. De Vitry, E. Colin and F. Tupin, "InSAR2InSAR: A Self-Supervised Method for InSAR Parameters Estimation," 2024 32nd European Signal Processing Conference (EUSIPCO), 2024, pp. 651-655, doi: 10.23919/EUSIPCO63174.2024.10715155.
C. Geara, C. Gelas, L. De Vitry, E. Colin and F. Tupin, "Extending InSAR2InSAR to Sentinel-1 Data," in IEEE Geoscience and Remote Sensing Letters, 2025, doi:10.1109/LGRS.2025.3558363.