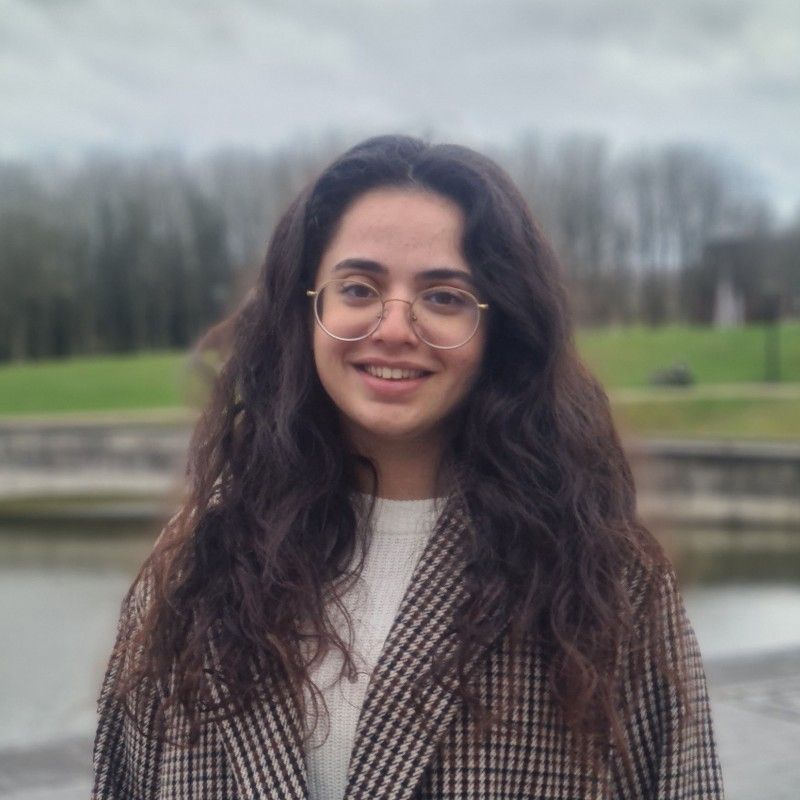
Carla Geara
Image Processing Engineer
We are excited to present InSAR2InSAR, a cutting-edge deep learning model that enhances the accuracy of Interferometric Synthetic Aperture Radar (InSAR) data by reducing speckle noise. This breakthrough improves forest biomass and canopy height estimations. It builds trust in nature-based projects by providing reliable verification (MRV) of their impact, while also allowing companies to better monitor their supply chains and natural assets.
In the face of accelerating climate change, reliable data on forest biomass and biodiversity are crucial for driving impactful conservation and climate action. Recognizing this need, Kanop is proud to announce the launch of our latest research innovation, InSAR2InSAR—a groundbreaking deep learning model designed to enhance the accuracy and clarity of Interferometric Synthetic Aperture Radar (InSAR) data. This development represents a significant leap forward in our mission to provide robust, science-based insights for sustainable land and forest management.
InSAR data has demonstrated significant potential for estimating forest structural parameters [1,2]. However, traditional remote sensing methods face a persistent challenge: speckle noise. This phenomenon, intrinsic to radar data, makes it difficult to exploit InSAR parameters. The InSAR2InSAR model tackles this issue head-on by reducing speckle noise, enabling clearer, more reliable data that can be used to monitor environmental changes with higher precision.
Unlike conventional models trained on synthetic noise-free data, InSAR2InSAR utilizes a self-supervised learning approach based on the Noise2Noise principle [3]. This technique allows our model to train directly on “noisy” data from real-world satellite images without requiring noise-free images, improving its effectiveness in practical applications. Through this process, InSAR2InSAR becomes a powerful tool for accurately estimating forest parameters from PolInSAR data (polarimetric and interferometric SAR).
This enhanced accuracy builds trust in nature-based projects by providing reliable verification (MRV) of their impact, while also allowing companies to better monitor their supply chains and natural assets.
This breakthrough would not have been possible without our research partners Télécom Paris and ONERA. Together, we are pioneering new standards for environmental monitoring, leveraging cutting-edge AI and remote sensing to drive meaningful progress in nature-based solutions.
The initial success of InSAR2InSAR on simulated data is only the beginning. Our team is now preparing to apply this model to real-world datasets from Sentinel-1, advancing our commitment to scientifically rigorous, field-ready applications for monitoring and protecting vital ecosystems.
As we continue developing tools for precise, impactful environmental monitoring, InSAR2InSAR exemplifies our dedication to science-backed innovation. For clients in the Voluntary Carbon Market and Nature-based solutions sectors broadly, this research opens new possibilities, offering a clearer path toward sustainable, climate-conscious decision-making.
[1] S. R. Cloude and K. P. Papathanassiou, “Three-stage inversion process for polarimetric SAR interferometry,” IEE Proceedings - Radar, Sonar and Navigation, Jun. 2003.
[2] G. Brigot, “Prédire la structure des forêts à partir d’images PolInSAR par apprentissage de descripteurs LIDAR.”
[3] J. Lehtinen, J. Munkberg, J. Hasselgren, S. Laine, T. Karras, M. Aittala, and T. Aila, “Noise2Noise: Learning Image Restoration without Clean Data,” in Proceedings of the 35th International Conference on Machine Learning, ser. Proceedings of Machine Learning Research, J. Dy and A. Krause, Eds. PMLR, 10–15 Jul 2018.